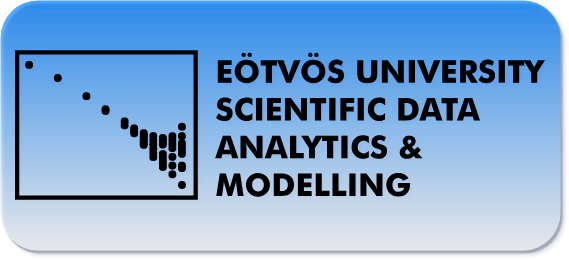
Using KNN algorithm to estimate photometric redshifts
- 12 mins02. Lab exercise, Supervised learning, KNN
- Implement naive K nearest neighbour regression as a function only using python and numpy. The signature of the function should be:
def knn_regression(x2pred, x_train, y_train, k=10):
"""Return prediction with knn regression."""
return y_pred
-
Apply the KNN regressor on photometric redshift estimation using the provided photoz_mini.csv file. Use a 80-20% train test split. Calculate the mean absolute error of predictions, and plot the true and the predicted values on a scatterplot.
-
Apply the KNN regressor on photometric redshift estimation using the provided photoz_mini.csv file. Use 5 fold cross validation. Estimate the mean and satndard deviation of the MAE of the predictions.
-
Repeat excercise (3.) with the KNN regression class from sklearn. Compare the predictions and the runtime.
-
Implement weighted KNN regression and apply it on the same data. Use 5 fold cross validation. Estimate the mean and satndard deviation of the MAE of the predictions. Plot the true and the predicted values from one fold on a scatterplot.
import numpy as np
import pandas as pd
import matplotlib.pyplot as plt
import time
dataset = pd.read_csv("photoz_mini.csv")
print(dataset.columns.values)
colors = ['u', 'g', 'r', 'i', 'z']
['Unnamed: 0' 'id' 'u' 'g' 'r' 'i' 'z' 'redshift']
# split u, g, r, i, z channels
sets = np.split(dataset.values[:,2:7], 5)
test = sets[0]
train = np.concatenate(sets[1:5])
# split redshifts
sets = np.split(dataset.values[:,7], 5)
test_redshift = sets[0]
train_redshift = np.concatenate(sets[1:5])
# get shapes
print(train.shape, train_redshift.shape)
print(test.shape, test_redshift.shape)
(800, 5) (800,)
(200, 5) (200,)
def knn_regression(x2pred, x_train, y_train, k=10):
y_pred = []
for dp in x2pred:
dists = np.array([np.sqrt(np.sum(np.square(dp-value))) for value in x_train])
ind = np.argpartition(dists, k)
neighbors_redshift = y_train[ind][:k]
y_pred.append(np.mean(neighbors_redshift))
return np.array(y_pred)
pred_redshift = knn_regression(test, train, train_redshift)
# Plot redshifts: predicted-valid
plt.figure(figsize=(10.,8.))
plt.scatter(pred_redshift, test_redshift, c="b")
plt.xlabel("Predicted redshifts")
plt.ylabel("Measured redshifts")
# Plot 45 degree line for grasping accuracy
x = np.linspace(np.min(pred_redshift), np.max(pred_redshift), num=1000)
plt.scatter(x, x, c="r", marker=".")
<matplotlib.collections.PathCollection at 0x7f85e100f898>
# get the mean squared error
MSE = np.sum(np.square(pred_redshift-test_redshift))/test_redshift.shape[0]
print("Mean squared error is %.5f" % MSE)
Mean squared error is 0.00766
# get the mean absolute error
MAE = np.sum(np.absolute(pred_redshift-test_redshift))/test_redshift.shape[0]
print("Mean absolute error is %.5f" % MAE)
Mean absolute error is 0.05062
def cross_validate(algo, dataset, splits=5, k=10):
MAEs = []
MSEs = []
start = time.time()
for i in range(splits):
# Sets for u, g, r, i, z indices
sets = np.split(dataset[['u','g','r','i','z']].values, splits)
x_test = sets[i]
x_train = np.concatenate([st for ind, st in enumerate(sets) if ind != i])
# Split for redshift
sets = np.split(dataset['redshift'].values, splits)
y_test = sets[i]
y_train = np.concatenate([st for ind, st in enumerate(sets) if ind != i])
y_pred = algo(x_test, x_train, y_train, k)
MAEs.append(np.sum(np.absolute(y_pred-y_test))/y_test.shape[0])
MSEs.append(np.sum(np.square(y_pred-y_test))/y_test.shape[0])
print("Mean MAE : %.10f" % np.mean(MAEs))
print("Standard deviation of MAE : %.10f" % np.std(MAEs))
print("Mean MSE : %.10f" % np.mean(MSEs))
print("Standard deviation of MSE : %.10f" % np.std(MSEs))
end = time.time()
print("The operation took %.5f seconds. " % (end-start))
cross_validate(knn_regression, dataset, splits=10, k=13)
Mean MAE : 0.0486281296
Standard deviation of MAE : 0.0036387536
Mean MSE : 0.0066927752
Standard deviation of MSE : 0.0017580858
The operation took 7.55900 seconds.
from sklearn.neighbors import NearestNeighbors
def sklearn_knn_regression(x2pred, x_train, y_train, k=10):
neigh = NearestNeighbors(n_neighbors=k)
neigh.fit(x_train)
y_pred = []
for indices in neigh.kneighbors(x2pred, return_distance=False):
y_pred.append(np.mean(y_train[indices]))
return np.array(y_pred)
sklearn_pred_redshift = sklearn_knn_regression(test, train, train_redshift)
# Plot redshifts: predicted-valid
plt.figure(figsize=(10.,8.))
plt.scatter(sklearn_pred_redshift, test_redshift, c="b")
plt.xlabel("Predicted redshifts")
plt.ylabel("Measured redshifts")
# Plot 45 degree line for grasping accuracy
x = np.linspace(np.min(sklearn_pred_redshift), np.max(sklearn_pred_redshift), num=1000)
plt.scatter(x, x, c="r", marker=".")
<matplotlib.collections.PathCollection at 0x7f85d6d90668>
cross_validate(sklearn_knn_regression, dataset, splits=5, k=7)
Mean MAE : 0.0498563174
Standard deviation of MAE : 0.0023512523
Mean MSE : 0.0071803898
Standard deviation of MSE : 0.0019106446
The operation took 0.01940 seconds.
# I rerun this here just to have the values below each other.
cross_validate(knn_regression, dataset, splits=5, k=7)
Mean MAE : 0.0498563174
Standard deviation of MAE : 0.0023512523
Mean MSE : 0.0071803898
Standard deviation of MSE : 0.0019106446
The operation took 6.69437 seconds.
All praise the skLearn package. I am AMAZED. :O The predictions are the same for 11 digits and the built in estimator is ~250 times faster than the one that I wrote from scratch.
def weighted_knn_regression(x2pred, x_train, y_train, k=10):
y_pred = []
for dp in x2pred:
dists = np.array([np.sqrt(np.sum(np.square(dp-value))) for value in x_train])
ind = np.argpartition(dists, k)
neighbors_redshifts = y_train[ind][:k]
neighbors_distances = dists[ind][:k]
y_pred.append(np.sum(neighbors_redshifts/neighbors_distances)/np.sum(1./neighbors_distances))
return np.array(y_pred)
weighted_pred_redshift = weighted_knn_regression(test, train, train_redshift)
# Plot redshifts: predicted-valid
plt.figure(figsize=(10.,8.))
plt.scatter(weighted_pred_redshift, test_redshift, c="b")
plt.xlabel("Predicted redshifts")
plt.ylabel("Measured redshifts")
# Plot 45 degree line for grasping accuracy
x = np.linspace(np.min(weighted_pred_redshift), np.max(weighted_pred_redshift), num=1000)
plt.scatter(x, x, c="r", marker=".")
<matplotlib.collections.PathCollection at 0x7f85d6d7f390>
cross_validate(weighted_knn_regression, dataset, splits=5, k=7)
Mean MAE : 0.0490389669
Standard deviation of MAE : 0.0023706854
Mean MSE : 0.0070522085
Standard deviation of MSE : 0.0019837564
The operation took 6.79338 seconds.
def sklearn_weighted_knn_regression(x2pred, x_train, y_train, k=10):
neigh = NearestNeighbors(n_neighbors=k)
neigh.fit(x_train)
y_pred = []
distances, indices = neigh.kneighbors(x2pred, return_distance=True)
for inds, dists in zip(indices, distances):
y_pred.append(np.sum(y_train[inds]/dists)/np.sum(1./dists))
return np.array(y_pred)
sklearn_weighted_pred_redshift = sklearn_weighted_knn_regression(test, train, train_redshift)
# Plot redshifts: predicted-valid
plt.figure(figsize=(10.,8.))
plt.scatter(sklearn_weighted_pred_redshift, test_redshift, c="b")
plt.xlabel("Predicted redshifts")
plt.ylabel("Measured redshifts")
# Plot 45 degree line for grasping accuracy
x = np.linspace(np.min(sklearn_weighted_pred_redshift), np.max(sklearn_weighted_pred_redshift), num=1000)
plt.scatter(x, x, c="r", marker=".")
<matplotlib.collections.PathCollection at 0x7f85d6ce3978>
cross_validate(sklearn_weighted_knn_regression, dataset, splits=5, k=7)
Mean MAE : 0.0490389669
Standard deviation of MAE : 0.0023706854
Mean MSE : 0.0070522085
Standard deviation of MSE : 0.0019837564
The operation took 0.02641 seconds.